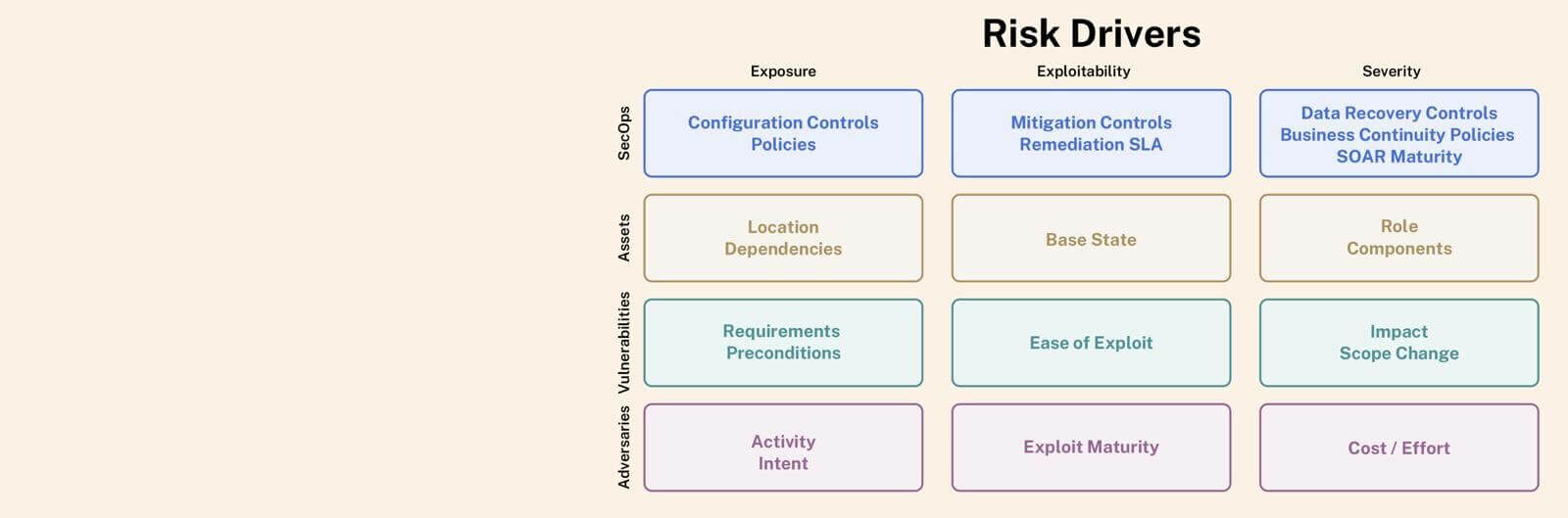
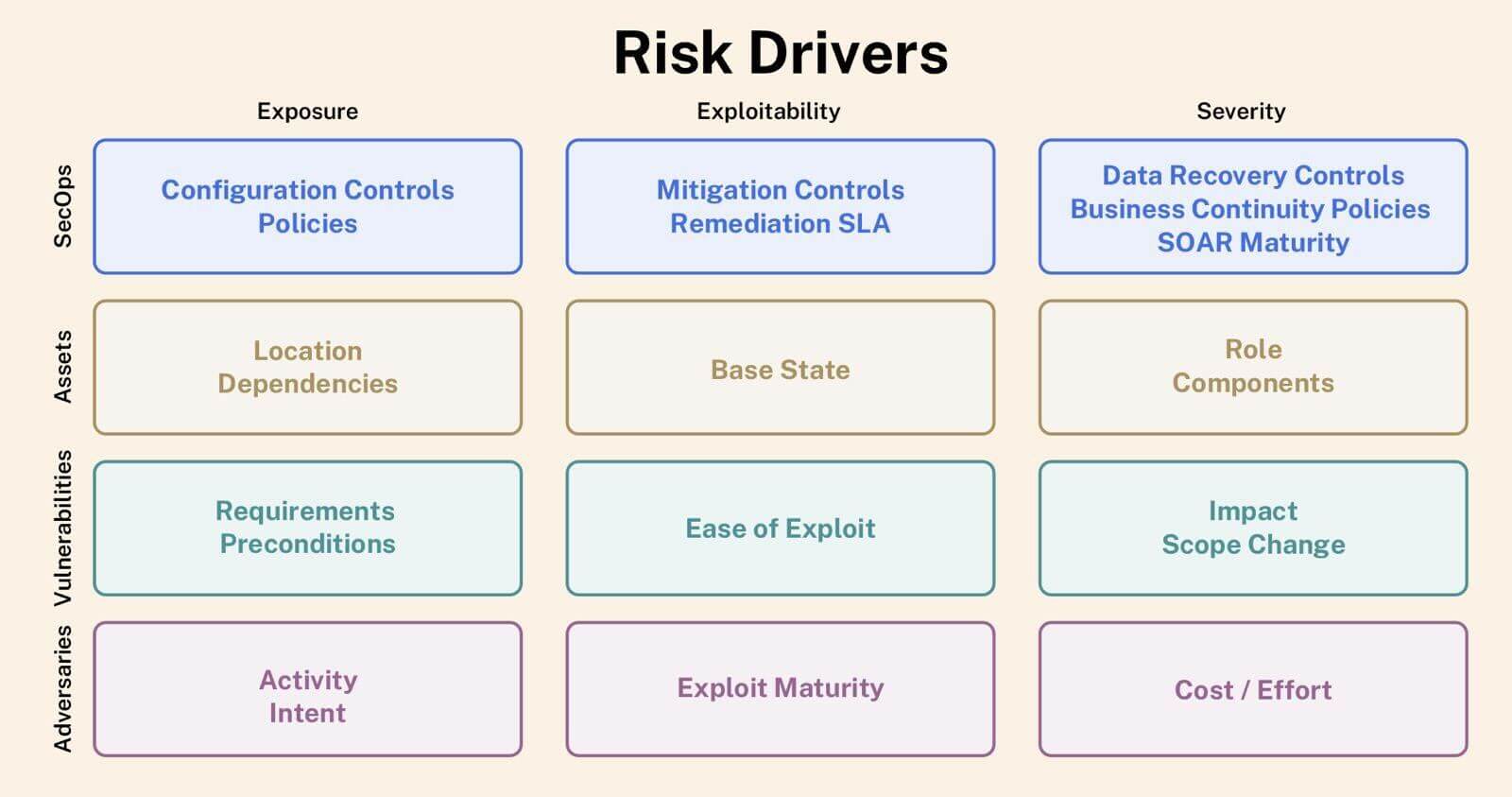
April 23, 2024
In this third and final post in the series on how AI plays a crucial role in cyber risk management, we will combine what we have learned in the previous posts on attack surface management and vulnerability prioritization. We will show you the role of risk quantification and how Balbix delivers data-driven risk quantification that is traceable and actionable.
Fundamentally, enterprise assets consist of devices, applications and users. In security, the impact of assets is measured by:
Building an asset inventory helps us not only understand our attack surface, but also dependencies among assets. As illustrated in the figure below, this lets us measure the impact of assets accurately using Bayesian principles, adjusting based on observed and inferred data.
With this impact estimation algorithm, we can arrive at a bottom-up data-driven impact assessment for every asset on the network. Furthermore, Balbix can analyze historical cybersecurity events and their associated costs to determine the cost of a breach for an entire enterprise.
This analysis can be performed at the level of business units, regions, or subsidiaries. It allows for precise control of impact across the organizational hierarchy using well-known and easily understood inputs. Additionally, our algorithm can incorporate custom assessments, in addition to historical data, to provide the most accurate impact estimate
Furthermore, Balbix can calculate the impact of assets on the network in monetary units (using local currencies) with both top-down and bottom-up estimates. We do this by using a graph-based inference algorithm which closely matches the data-driven bottoms-up approach.
The algorithm also considers constraints from our top-down impact assessment based on historical events.
They’re the things that make an organization vulnerable to risks. Three main factors determine how risky a vulnerability is:
The entities that influence these risk drivers are as below.
In an ideal state, an asset is not exploitable. However, on the basis of its business, it has a severity, or impact. The functionality it supports might also require it to be contributing to exposure of risk. This is depicted in the figure below, along with some examples of factors that contribute to the risk drivers.
The existence of vulnerabilities enables exploitation, and therefore they are the first contributors to exploitability. Vulnerability attributes also contribute to exposure and severity.
The threat of exploitation from adversaries influences all the risk drivers, and the factors are illustrated below.
The maturity of security operations also influences the risk drivers. A strong proactive strategy reduces exposure, and a robust protection strategy can mitigate exploitability and severity.
By considering the three risk drivers across the four entities described in the previous section for every vulnerability identified on the network, Balbix arrives at a completely data-driven, inspectable and actionable risk quantification. We employ Bayesian probabilistic graphical models to factor every data observation towards how they influence risk and assess the risk exposure from each vulnerability instance. It is aggregated to any collection – the risk from each asset component, the risk from each asset, the risk from any arbitrary group of assets, all leading to the risk at the level of the enterprise.
With the quantification of risk at all granularities highlighted above, the importance of each risk source can be quantified. We can also answer questions such as –
Noting that cyber risk quantification is not an end in and of itself, the ability to ask and answer these questions is crucial in realizing the virtuous cycle of risk reduction from the various security operations run by enterprise security teams.
Moreover, as a consequence of this approach that lends itself perfectly to actionability, Balbix can further use AI to recommend the next best steps for any user based on their persona and purview.
To learn more, request a demo.